Summary
Energy inefficiency is a serious issue in the Vietnamese industry, which is the sector consuming the most energy in Vietnam. This article adopts stochastic frontier analysis (SFA) to evaluate the factors that influence the energy inefficiency in Vietnamese enterprises. Under the evaluation for the tile industry, the empirical results indicate that there are many factors having significant impacts on energy inefficiency, including fuel type, ownership structure, size of firms, labour quality, energy price and import activities. Related to the fuel type indicator, this article discovers that the level of energy inefficiency in firms using gases is 1.258 times lower than in firms using other fuels. That is the obvious evidence for the better performance of gas consumers in term of energy efficiency.
Key words: Energy efficiency, stochastic frontier, SFA, gas consumers, Vietnamese industrial sector, industry energy efficiency.
1. Introduction
The industrial sector plays an important role in the development of the Vietnamese economy, contributing the largest share (38%) of the country’s GDP [1]. Corresponding to the contribution rate of GDP, the industrial sector accounts for the highest proportion of energy consumption (38.2%) in total energy demand [2]. Nevertheless, one can see that the inefficient energy usage in the industrial sector is one of the main reasons for overall energy inefficiency in Vietnam. Table 1 implies that, to generate USD 1,000 of GDP, the Vietnamese industrial sector consumes the highest amount of energy in comparison with many countries. More precisely, energy use per GDP of the Vietnamese industry is 3 times higher than the average energy consumption of the world. It is also 1.7 times higher than the average level of other developing countries, including Thailand, China, Malaysia and Indonesia.
According to the industrialisation strategy of the Vietnamese Government, it is expected that the Vietnamese economy and the energy consumption will maintain similarly rapid growth rates up to 2020. Therefore, a possible consequence is that Vietnam may experience energy shortage soon or later. Reduction of the energy inefficiency, which is claimed as the national target by the Vietnamese government, becomes an urgent mission. It is necessary to focus on minimising the energy inefficiency in the industrial sector, especially in industries consuming high energy, such as tile production, steel industry, or cement production. Hence, the goals of this article are to evaluate the energy inefficiency and to identify the potential factors causing energy inefficiency in the industrial sector. The approach and the results of this article also can help the Government to find better strategies for improving energy efficiency.
Table 1. Comparison of energy consumption per GDP by industry
Furthermore, the Vietnamese government is pursuing the goal of sustainable development, in which economic development must be accompanied by energy security and environment protection. It implies that cleaner fuels like LPG or NG are more encouraged than the other types. Thus, this article also aims to evaluate the difference in energy efficiency between companies consuming gases (including LPG and NG) and those consuming other fuels.
To the best of our knowledge, there is hardly any published study to assess the factors affecting energy efficiency in the Vietnamese industry. Therefore, this article is a significant contribution to an overlooked research field in energy economics in Vietnam. In addition, empirical researches assessing energy efficiency can be classified into three main approaches: energy intensity, data envelopment analysis (DEA) and stochastic frontier analysis (SFA). While there are numerous studies applying energy intensity or DEA to measure energy inefficiency, thereareonlyfewstudiesapplying SFAandallmainlyfocus on country/region level. Therefore, another important contribution of this article is to explore the performance of SFA in energy economics with firm-level data.
The remaining parts of this article are organised as follows. Section 2 describes the methodology employed in this article. Section 3 explains the estimation and the data/variables collected to implement the model. Section 4 provides the quantitative analysis. Section 5 discusses the policy implications of the empirical results. We summarise our main findings in Section 6.
2. Analytical framework
Boyd [3] points out SFA can evaluate energy efficiency either through a normal statistic function or through production function. The former application is adopted to evaluate energy efficiency for OECD countries and for US residents by Filippini and Hunt [4, 5]. The later approach, so- called energy input distance function, is employed by Zhou, Ang and Zhou [6], Honma and Hu [7] and Lin and Long [8]. Following Zhou, Ang and Zhou [6] and Lin and Long [8], this article employs the energy input distance function approach with the assumption of Translog function.
Technology inefficiency and input distance function
All production processes are understood as processes to transform inputs into outputs under specific technologies. The transformation relationship between inputs and outputs can be described by a mathematical formula, so-called the production function. A production function can be written in the form of F(X, Y) = 0, where X is an M dimension non-negative input vector and Y is an N dimension non-negative output vector. In case of production with only one output, the production function will be Y = f(X1, X2, ..., Xn) where the function f(.) represents a technology governing the production process.
Based on technology of production, there are several types of production function adopted in economic analysis, in which Cobb-Douglas and Translog production functions are the most well-known. The Cobb-Douglass function form is described in normal form as
or in natural logarithm form as
where the parameters βi satisfy that
and
for all
and
(A is a constant number). The Translog function form can be explained as
where Y is output of production
, Xi is inputs of production, are parameters and
.
In the literature of production function, the relation between inputs and output is assumed as the frontier of attainable production set. It means, with given inputs Xi, a firm always produces the maximum possible output. Meanwhile, given output Y and all other inputs Xj (where j ≠ i), the value of input Xi is always determined at the minimum level. However, in the production efficiency literature, that assumption will be relaxed. Thus, with given inputs, firms may produce less than the maximum possible output(s), or firms may need more than the necessary level of inputs to produce the given output. In those cases, firms have technical inefficiency.
Figure 1 provides a graphic demonstration of a technically inefficient production. Point A that locates under production frontier Y = f(X) represents that a firm is not technically efficient. The inefficiency of firm A can be explained in two ways based on the input-oriented or the output-oriented measure. From the view of input-oriented measure, the observed output level (Y0) can be technically produced consuming fewer inputs (X* instead of X0). In the graph, firm A can become efficient by reducing inputs and move to point C on production frontier. The distance AC indicates the wasted inputs due to technical inefficiency and it is the basis to measure input-oriented (IO) technical inefficiency. More specifically, IO technical inefficiency is measured by which is the proportion of inputs that firms can reduce to become efficient. From the view of output-oriented measure, there is a possibility to technically attain higher level of output (Y* instead of Y0) for given inputs (X0), so that firm A can move to point B on the production frontier. The gap AB in the graph implies the output loses out because of technical inefficiency and it is the basis to measure output- oriented (OO) technical inefficiency. More precisely, OO technical inefficiency is measured by which is the proportion of output that firms can increase to become efficient.
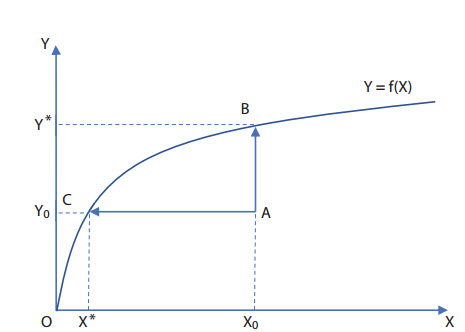 |
Figure 1. Input-oriented and output-oriented technical efficiency
In mathematics, the input-oriented inefficiency can be explained as
In that equation, uX is input-oriented inefficiency, Dl(.) ≥ 1 is the input distance function, homogenous of degree one of inputs vector x, A(Y) is the required set of inputs that is feasible to produce the observed outputs Y. Therefore, a firm will be considered as efficient if value of Dl is equal to 1.
Energy input distance function
Let E is vector of energy demand to produce observed vector of outputs Y for given vector of non- energy inputs X, the energy input distance function is stated that:
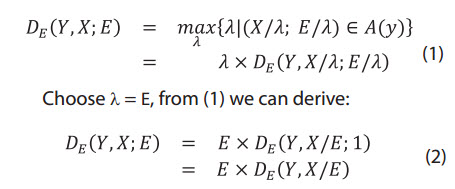
More specifically, for a general production with two non-energy inputs being capital (K) and labor (L), take a natural logarithm for both sides of equation (2) we have:

Let as energy inefficiency in log form of input distance function, equation (3) can be re-written:

It is possible to expand equation (4) with assumption of Cobb-Douglas production function form or Translog production function form as in equation (5) and equation (6), respectively.
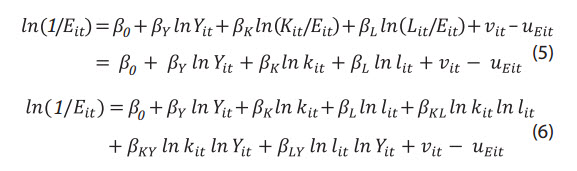
Where v is statistical error,
is measured by the fraction between total capital, K and energy consumption, E. Variable is measured by the fraction between total labour, L and energy consumption, E. The non-negative energy inefficiency, uE is measured in log form of input distance function uE Ξ lnDE(Y, K, L; E), where the higher value of uE leads the lower value of ln(1/ Eit) and it is corresponding to the higher energy consumption, E.
Thereafter, energy inefficiency can be determined by adopting standard SFA estimation methods to estimate equations (5) and (6). Furthermore, this article also evaluates the factors affecting energy inefficiency of firms through the equation uEit = u(zit) where zit is a vector of exogenous variables such as energy price, the enterprise size or the ownership structure. Both of above goals can be achieved simultaneously by the one-step estimation approach that is widely applied in recent researches. The detail of the estimation method will be described as in the next section.
3. Estimation and data
Estimation
Since the development of the estimation method for panel data by Pitt and Lee [9], the literature of SFA has steadily improved with several methods. This article follows the procedure of Battese and Coelli [10], which is the most adopted method in empirical researches, to evaluate the energy inefficiency via energy distance function. In addition, this article follows suggestion of Lin and Long [8] to setup model with assumption of Translog production function form. The empirical model can be expressed as:
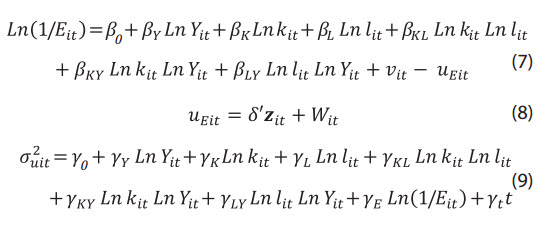
(10)
In this article, the Translog setting will be adopted Equation (7) is energy frontier equation that is identical with equation (6).
is energy inefficiency,
is the statistical error. Equation (8) is inefficiency effects equation where zit is vector of exogenous variables (will be clarified in Section 3.2).
is random variable truncated at point z , where is vector of parameters which may include constant term. Equation (9) is to control heteroscedasticity in UEU, where is variance of . Equation (10) is to control heteroscedasticity in, where is variance of. Variable t is time variable (if any) and are vectors of parameters. The parameters of all equations are estimated simultaneously by programme developed by Kumbhakar, Wang, and Horncastle [11] on the platform of Stata software.
Data
In order to provide an in-depth analysis and based on availability of the data, we narrow down the scope of the study focusing on the tile industry for our empirical analysis. The data we used in this article is the latest firm- level panel data of the Vietnamese tile industry, which covers 574 firms throughout the territory of Vietnam over the period of three years. The entire data is collected from the Business Survey conducted by the General Statistics Office of Vietnam annually. For the estimation of energy frontier equations, we use four panel series which are: (1) aggregate energy consumption, (2) total capital, (3) total labour that participates in the production process, and (4) the value added. Meanwhile, for inefficiency effects equations we include six variables: (1) fuel type, (2) ownership structure,(3) enterprise size, (4) average price of energy, (5) quality of labour, and (6) import activity.
Variables in energy frontier equation
Production process in tile companies consumes assorted energies including electricity, coal, gasoline, diesel, LPG and natural gas. In addition, the combination of energies varies from firm to firm. Therefore, we adopt the widely accepted approach that aggregates energy consumption which is the summation of the heating value of all consumed energies for producing purpose. Aggregate energy consumption, E, is measured in billion British thermal unit (billion Btu).
Firms’ total capital are collected as variable K, which is measured in billion Vietnamese dongs (billion VND) based on the year 2010. Variable k, then, is derived by fraction between K and E, before taking natural logarithm to fit with setup of the model mentioned above.
Total labour that participates in the production process is denoted by L and is presented in number of employees. We take the fraction between L and E to derive variable l, then take natural logarithm to be suitable with the setup of the SFA model.
The value added of tile production is collected as variable Y, before taking natural logarithm to fit with the setup of the SFA model. Variable is measured in billion Vietnamese Dong (billion VND)i.
The following discussions will explain the potential factors which might affect the energy inefficiency in the Vietnamese tile industry, which includes fuel type, ownership structure, enterprise size, price of energy, labour quality and import activity.
Fuel type
The most important purpose of this article is to determine the difference in energy efficiency between firms using conventional fuels (e.g. coal, diesel) and firms using cleaner energies (e.g. LPG or NG). It is an well-known fact that use of gases leads to higher performance than use of other fuels. However, under the same conditions of the production (same amount of output, same enterprise size, and quality of labour, etc) the difference in energy efficiency of using gas and of using other fuels is still ambiguous.
In order to assess the effect of fuel type on energy inefficiency, this article adopts the dummy variable type, where type equal to 0 represents firms using gases and type equal to 1 is for production without gases.
Ownership structure
Many tile companies have state-owned share in capital, and it is understood that state-owned firms are associated with inefficiency [12]. In order to capture that impact, this article uses the dummy variable gov_sh as an exogenous, where state-related firm has gov_sh equal to 1 and otherwise, gov_sh is equal to 0.
Enterprise size
The large-sized firms are usually considered more efficient than smaller ones since they are able to purchase better equipment and to recruit more productive employees [8]. However, since large-sized firms have more advantages to access energy resources, they may have less incentive to consume energies efficiently than smaller ones. This article evaluates the difference in energy efficiency between different sizes of firms through dummy variable size. The large-sized firms (represented by size equal to 0) and small and medium-sized firms (SMEs, represented by size equal to 1) are defined in the Vietnamese Government’s Decree No. 56/2009/ND-CP. i There are 3 out of 1,722 observations in data set which have negative or zero value added and will be automatically excluded when we take log. Since they are very small in number, they do not affect the results of estimation.
Average price of energy
The price of energy is an important factor in energy efficiency analysis, where the higher the energy price is, the higher energy efficiency might be. Due to the facts that tile firms use many types of energies simultaneously and there is no competitive energy market in Vietnam, the use of unit price becomes inappropriate. Thus, this article employs the average price that is calculated by the division of total cost for energies and aggregate energy consumption determined before. Thereafter, they will be transformed to natural log form and be denoted as lp.
Quality of labour
Economists accept that the quality of labour is conducive to improving production efficiency. This article employs the average salary, lql, to investigate the impact of labour quality on energy inefficiency, in which higher average salary implies higher quality of labour. Data of firms’ salary are measured in million Vietnamese dongs per person and they are transformed into natural logarithm form as lql.
Import activity
It is believed that firms having to import materials/ fuels will have more incentive to control its production to be more efficient than other firms. This article creates a dummy variable im to investigate the difference in energy inefficiency between firms having import activities (im equals to 1) and others (im equals to 0). Statistical summary of the above variables is given in Table 2.
4. Quantitative analysis
The estimation results displayed inTable 3 indicate that all models are valid with high significance in parameters. In energy frontier equation, the estimated coefficients are strongly significant at the 0.1% significance level. Besides that, in inefficiency effects equation, almost estimated coefficients are highly significant at level of 5% or even at level of 0.1%.There is only one exception - the coefficient of im is only significant at the level of 27% in case of Translog function. Due to most of materials for the tile industry can be supplied by the domestic market, not many companies have import activities. Thus, the significance level of 27% and the appropriate sign of coefficient (negative sign) are noticeable results to convince that we should not drop it out of model.
Table 3 also shows that there is heteroscedasticity in inefficiency term but no heteroscedasticity in statistical error. While a few coefficients in variance equation of inefficiency term are not really significant, other coefficients are highly significant at level of 0.1%, so that circumstance is still acceptable.
It is noteworthy that the parameters of exogenous variables in the inefficiency effects equation displayed in Table 3 are valueless for economists. The reason is that they are coefficients of pre-truncated distribution of inefficiency uEit which has truncated distribution, so that they could not explain the real effect of exogenous variables on uEit. In order to understand the real impacts of explanatory factors on energy inefficiency, this article calculates the partial effect (or marginal effect) of each factor via . The formula and the command in Stata to compute the marginal effect of kth factor zk evaluated at sample mean ) is provided by Kumbhakar, Wang, and Horncastle [11]. Those results are shown in Table 4.
Table 2. Descriptive statistics for the variables
Note: *: significant at level of 0.05; **: significant at level of 0.01; ***: significant at level of 0.001
Table 3. Results of estimation
Table 4. Avezrage partial effect of exogen6ous v(auriabl)e/s6on(z )
5. Discussion
Impact of fuel types on energy inefficiency
The coefficient of type on energy inefficiency is positive with significance level of 0.1%, implying higher inefficiency in the tile enterprises using conventional fuels (e.g. coal, diesel) than in gas consuming enterprises.
More specifically, the results in Table 4 indicate that the energy inefficient gap between firms using conventional fuels (e.g. coal, diesel) and firms using gases is
Thus, we can derive 
This result shows that the level of inefficiency in firms using conventional fuels is higher than that in firms using gases by 1.258 times. In other words, firms using gases are 1.258 times more energy efficient than other firms.
It is remarkable that the emission of gas consumption is much lower than the emission of other fuels. Figure 2 indicates that natural gas and LPG are the fuels with the least CO2 emission factor. Natural gas and LPG emit respectively 0.2kg and 0.23kg CO2 for each kWh (equivalent) consumed while other type of fuels, which are used in the tile production, release from 0.27kg to 0.36kg CO2.
According to the database, there are only 40 out of 574 enterprises using LPG or NG in their production. Thus, if the Vietnamese Government would like to achieve sustainable development goals, one thing that the industry sector and the tile industry, in particular, can help is to encourage as many firms to use environmentally friendly energy such as NG and LPG as possible.
Figure 2. CO2 emission by type of fuels (kgCO2/kWh) [13]
Of course, the tile producing firms may be concerned about the cost of technology conversion and the fact that gas price is higher than the price of conventional fuels. The economic efficiency of technology conversion is not a scope of this article and should be considered carefully in another study. However, based on the data of the tile industry that is used in this article, the average cost firms using gases need to pay for each million Btu energy consumption is about VND 0.412 million, while that number in firms using conventional fuels is VND 0.74 million. That comparison implies that using gases in production is considerably cheaper than using other fuels. It is also noteworthy that the quality and the productivity of tile production using gases are higher than those of production using conventional fuels. Therefore, a proportion (or all) of conversion cost and energy price difference may be absorbed by the spread of products’ price.
Impact of ownership structure on energy inefficiency
The coefficients of dummy variable gov_sh are significantly positive, implying that state-related firms (gov_sh = 1) are more inefficient than non-state ones (gov_sh = 0).
From Table 4, we can derive the ratio of input distance function between a state-related firm and a non-state firm
as
to prove that the energy input distance of state-related firms is 1.313 times higher. It implies the ratio of energy consumption to be reduced in state-related firms is 31.3% higher than that ratio in non-state firms. Therefore, in order to increase energy efficiency, the tile industry may think about reducing the number of state-related firms by equitisation or privatisation.
Impact of enterprise size on energy inefficiency
The results from Table 3 show that enterprise size has significantly negative impact on energy inefficiency in Vietnam. More specifically, from Table 4 we can evaluate the ratio…. It means the proportion of energy consumption which needs to be cut-off in SMEs is lower than that in large firms by 35.4%.
That result is contrary to circumstances in many other countries where large firms have an advantage of scale to be more efficient than the others. The reason may be (i) many of the Vietnamese large companies have many employees but not all of them are productive (tobe discussed below), or (ii) many big companies having huge capital are state-related companies (as discussed above), or (iii) the quality of management. The sources of inefficiency vary from case to case so it is not possible to propose a comprehensive solution for all.
Impact of labour quality on energy inefficiency
The results in Table 3 indicate that labour quality has a negative impact on inefficiency. Firms that have higher quality of labour, which was implied by higher average salary, have lower level of inefficiency. In addition, Table 4 shows that for firms having higher salary (e.g. 10%) will have lower the proportion of energy that needs to decrease (e.g. 2.184%).
In order to improve energy efficiency in the Vietnamese tile industry, firms should consider improving their labour quality, especially with focus on improving the quality of current employees.
Impact of energy price on energy inefficiency
The variable lp, as show in Table 3, has a negative effect on energy inefficiency. However, according to the marginal impacts recorded in Table 4, that effect is pretty small. In detail, in response to 10% increase in energy price, the energy input distance (or the proportion of consumed energy needed to reduce) decreases only 0.566%.
This phenomenon is actually not something unexpected. It is remarkable that the Vietnamese energy market is not competitive, so that the difference in energy prices here is caused by 2 main factors. Firstly, big contracts made by large firms will have more discounts so that the average cost will be lower than that in smaller firms. Secondly, firms using conventional fuels (e.g. coal, diesel…) have smaller average cost than firms using eco- friendly fuels such as natural gas and LPG. Because the impacts of the firm size and the use of conventional fuels are already captured in the model, the impact of price on energy inefficiency is attenuated.
Nevertheless, energy price is still important and should be considered in the inefficiency effects equation because when the Vietnamese energy market becomes more competitive, lp will show more obvious impact on energy efficiency/inefficiency.
Impact of import activities on energy inefficiency
Partial effect of variable im is considerably small in Table 4. Besides, the t ratio of that factor reported in Table 3 implies it is not really significant (only significant at level of 27%). However, since most of materials for the tile industry can be supplied by the domestic market, not many companies have import activities. The descriptive statistic in Table 5 displays that only 116 out of 1,722 observations have value of im equal to 1 (i.e. firm has import activities). Thus, the significance level of 27% and the appropriate sign of coefficient (negative sign) are noticeable results to convince we should not drop it out of the model.
Table 5. Statistical summary of variables for import activities
It is possible to claim that import activities have a negative impact on the energy inefficiency. Therefore, in order to improve energy efficiency, the Vietnamese tile industry may encourage firms to be more dynamic in the import-export market.
6. Conclusion
This article implements stochastic frontier analysis to evaluate energy technical inefficiency for firm-level panel data of the Vietnamese tile industry and the factors affecting that inefficiency follows the procedure of Battese and Coelli [10]. The empirical results figure out the significant impacts of exogenous factors on energy inefficiency, including fuel types, ownership structure, enterprise size, labour quality, energy price and import activities. These findings can help the Government have better evidences for considering the strategies to achieve the goals of sustainable development. Therein, the Government may think about some solutions, such as fuel conversion, equitisation or privatisation of state- related companies, and improving the quality of the labour force.
Concerning the influence of fuel types on energy efficiency, this article discovers the difference between firms consuming gases in production and firms using other fuels. More specifically, although there are only 40 out of 574 companies in the tile industry that utilise gases, the energy efficiency of these firms is 1.258 times better than that of the remaining firms. A comparison of energy costs shows that gas consumers actually need to pay only VND 0.412 million/million Btu energy on average, while consumers of other fuels have to pay almost double that amount (VND 0.74 million/million Btu on average). Thus, although there may be restrictions about conversion cost, the Vietnamese tile industry should be encouraged to convert to using environmentally friendly energy to achieve energy efficiency, less emission and higher productivity.
This article has some important contributions for stochastic frontier analysis in the energy sector, as well as for researches on energy inefficiency in Vietnam. However, certain restrictions still exist in this article. For example, the scope of time in 3 years is relatively short, or the study does not cover some new issues in the SFA such as scaling property of dataset. In addition, there are some potential for further studies as discussed below.
Firstly, this article uses input distance function for energy inefficiency with firm-level data. There are several researches applying the same procedure for province- level data. Thus, other authors in the Vietnamese energy market can conduct studies with province-level data, because energy efficiency analysis is still really new in Vietnam.
Secondly, there is a newer model proposed by Green [14, 15] which is more flexible than Battese and Coelli [10], but it requires a panel data set for at least 10 years. With current statistic data, it is impossible to satisfy that condition. However, in the next few years there will be no more constraints and that will be an opportunity to apply that more advanced model.
Furthermore, this article focuses on the tile industry due to the limitation in statistic data of other industries such as steel industry, cement industry. When longer panel datasets become available, it is possible to conduct studies on those fields and people will have a comprehensive view about energy inefficiency throughout the industrial sector.
Acknowledgements
The author would like to thank Professor Chun- Hung Kuo in the International University of Japan for his continuous support in conducting this research. This work was supported by the Japanese International Cooperation Centre (JICE).
References
1. World Bank. World development indicators 2017. 2017.
2. International Energy Agency. Energy balances of Non-OECD countries 2017. 2017.
3. Gale A.Boyd. Estimating the distribution of plant- level manufacturing energy efficiency with stochastic frontier regression. US Census Bureau Center for Economic Studies Paper No. CES-WP-07-07. 2007.
4. Massimo Filippini, Lester Charles Hunt. Energy demand and energy efficiency in the OECD countries: A stochastic demand frontier approach. Energy Journal. 2011; 32(2): p. 59 - 80.
5. Massimo Filippini, Lester Charles Hunt. US residentialenergydemandandenergyefficiency: A stochastic demand frontier approach. Energy Economics. 2012; 34(5): p. 1484 - 1491.
6. P.Zhou, B.W.Ang, D.Q.Zhou. Measuring economy- wide energy efficiency performance: A parametric frontier approach. Applied Energy. 2012; 90(1): p. 196 - 200.
7. Satoshi Honma, Jin-Li Hu. A panel data parametric frontier technique for measuring total-factor energy efficiency: An application to Japanese regions. Energy. 2014; 78: p. 732 - 739.
8. Boqiang Lin, Houyin Long. A stochastic frontier analysis of energy efficiency of China's chemical industry. Journal of Cleaner Production. 2015; 87: p. 235 - 244.
9. Mark M.Pitt, Lung-Fei Lee. The measurement and sources of technical inefficiency in the Indonesian weaving industry. Journal of Development Economics. 1981; 9(1): p. 43 - 64.
10. George Edward Battese, Tim J.Coelli. A model for technical inefficiency effects in a stochastic frontier production function for panel data. Empirical Economics. 1995; 20(2): p. 325 - 332.
11. Subal C.Kumbhakar, Hung-Jen Wang, Alan P.Horncastle. A practitioner's guide to stochastic frontier analysis using stata. Cambridge University Press. 2015.
12. Martin Painter. The politics of economicrestructuring in Vietnam: The case of state-owned enterprise "reform". Contemporary Southeast Asia. 2003; 25(1): p. 20 - 43.
13. Vietnam Petroleum Institute. Vietnam Petroleum Institute’s database. 2017.
14. Willam Greene. Fixed and random effects in stochastic frontier models. Journal of Productivity Analysis. 2005; 23(1): p. 7 - 32.
15. Willam Greene. Reconsidering heterogeneity in panel data estimators of the stochastic frontier model. Journal of Econometrics. 2005; 126(2): p. 269 - 303.